I wrote the original version of this post over 4 years ago. In revisiting this it is interesting to note that not much has actually advanced in the field. Yes, there have been more products and tools developed to apply FAIR or FAIR-like quantitative methods - some successful and some less so, usually indexed on the degree of effort it takes to set up the tooling to get more value out than you put in.
As with other areas of risk there’s a Heisenberg-like quality to much of the approaches. That is the act of measuring often changes the situation, often positively. Although the Breaking Bad use of the term Heisenberg might also apply given the mix of confusion and euphoria that often results from excess use of risk quantification.
There have also been significant advances in the world of cyber-risk insurance particularly in insurers fine tuning their actuarial models, having more data available from the insured parties, and from industry sources like research teams, intelligence units and some cyber scoring services. Some cloud providers and cybersecurity companies have also partnered to stimulate capacity and provide risk-adjusted pricing.
But overall, I think there has been the most progress in the effective use of data, analytics and visualization in specific domains like managing software vulnerability risk, extended supply chain risk through to identity and access management (human and non-human). We’ve also seen tremendous progress in the use of analytic techniques in posture management and continuous controls monitoring, especially coupled with risk and threat ordered prioritization of responses in operations (vulnerability management or detection & response). We should feel good about this even though the “Holy Grail” of effective and precise macro-scale risk quantification still eludes us. There has also been some useful application of AI techniques from deep learning, graph neural networks, and some use of LLMs to provide decision support for a number of risk management activities.
It’s with this backdrop that I think much of the point of the original post still stands - that risk quantification, in any field, is not an end in itself. It exists to compel some action. That action might be to drive decisions or simply to inform other analysis which in turn leads to some action. That great risk manager (and Stoic philosopher) Epictetus (“It is impossible for a man to learn what he thinks he already knows.”) said it best:
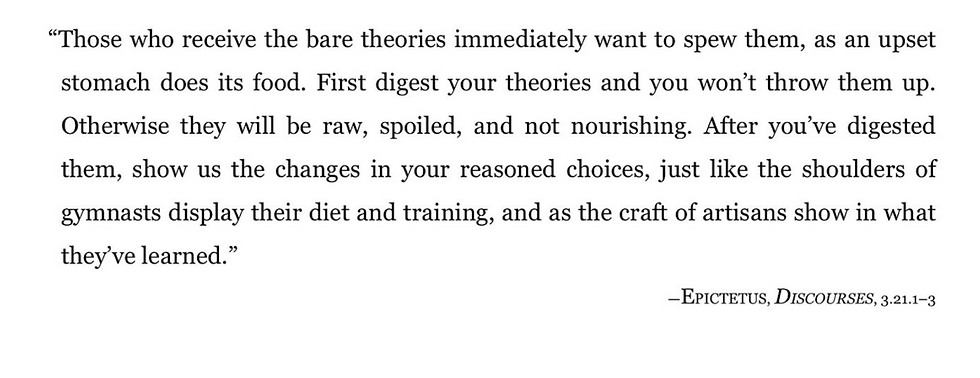
For as long as I’ve been involved in security and then more broadly enterprise risk management I’ve seen many positive developments and false starts. From this I continue to observe the following apparent truths:
1. Risk Quantification & Risk Communication - Big Difference
Risk quantification and risk communication are two different disciplines but they’re often confused. Most criticism of risk quantification is actually criticism of risk communication techniques that have been dressed up or misinterpreted as risk quantification. Pick your tool and use it in the right way. There are a variety of quantification mechanisms ranging from basic counting metrics, Bayesian network models, game-theoretic analysis, to techniques to model, for particular scenarios, the frequency distribution of potential events coupled with the severity distribution if those events occur. This is then used to compute a loss distribution to make more formal risk tolerance decisions. FAIR is a good example of this latter technique but has the added advantage of a well-worked ontology and supporting practice to aid in analytical rigor - and an increasing set of products and services that make this tractable.
One thing is common to all these techniques, they have to be interpreted and communicated, even to sophisticated audiences. It’s fine for the results of such quantification to be overlaid on grids, to be translated into ratings (High, Medium, Low) as long as the medium of doing that doesn’t lose the message.
I would argue that simple pseudo-quantification techniques like Risk = Threat x Vulnerability are mostly flawed, quite simply because the inputs to such a simple equation can never accurately encapsulate what is going on in a particular situation and it presents an overly simplistic view of risk. For example, Risk (0.3) = Threat (0.5) * Vulnerability (0.6). What does this even mean? (I’ve seen this actual example in an industry report). The other problem to watch out for is the naive assignment of potential monetary losses to metrics developed without using an appropriate loss distribution model.
But overall, the best risk modeling and quantification approaches are those that surprise even the intuition of the risk managers. I remember in one organization the use of a Bayesian Network to model the “network” of controls that contributed to a particular risk management goal - in this case a certain type of insider risk mitigation. There were about 10 inter-linked controls each with their own characteristic failure distribution (in turn modeled from actual event history) that when brought together in the network model, and subject to a Monte-Carlo simulation, revealed an unexpected dependency on a small number of controls - especially on one particular control. I don’t know why we were surprised that a Pareto distribution appeared here showing that 80% of the risk mitigation came from 20% of the controls. But we were. Even more concerning was the intuition that this wasn’t the most important control and thus was underinvested. We corrected that as a result of this modeling. No big fan-fare, no big Board presentation, just the routine day to day work of a good risk team. Perhaps this is going on in more places than we all think.
2. Remember Risk = Hazard + Outrage
In my experience, this is the most important equation in risk management. No matter how cooly and calmly we quantify what hazard we are subject to it can still be overwhelmed by outrage. Outrage from customers, governments, regulators, media, auditors and one’s own Board and management in turn influenced by that external outrage. You may well be thinking, isn’t this just reputational risk that should be included in the hazard. Sometimes, yes, a lot of times no.
This all comes from Peter Sandman’s excellent body of work, while being more about crisis communication and precaution advocacy it does have a myriad of lessons in how to communicate risk to drive the right outcomes.
3. Experience and Judgement Eats Data (alone) for Breakfast
Risk is managed by experienced people with judgment using data, not by the data alone. The world is littered with failures across many disciplines where the numbers (models or other techniques) suggested a course of action that was, on balance, actually wrong. Many of the mature uses of risk quantification in spaces from safety and hazard analysis, pharmacology to financial risk all have the same theme in common, that they rarely rely on just one number or output from one model. What they most often do is build decision making processes that are informed by multiple streams of data. Risk managers spend a lot of time looking for contradictions in the data that would indicate some underlying problem that needs further investigation. Risk managers also spend a lot of time looking for common themes across the data that increases the confidence level for a subset of potential courses of action. Consider a very simple example, let’s say you have a model that is aiming to predict security incidents in your supply chain and it asserts say a 0.2 likelihood of some security breach from a group of 20 of your most critical vendors in the next 12 months, but an adjacent model predicts a 0.8 likelihood in the same group for a reliability/error event. Both of those might be correct (or reasonable enough) but it certainly warrants a deeper dive in the underlying data to look at why this is divergent when, using your judgment, you know that the underlying controls for both outcomes can be correlated.
So, when working with risk quantification, think about how it is used and challenged inside a decision making process. There are some stark illustrations in many risk disciplines, I recall (in financial risk) during the 2008 financial crisis that the organizations who did well went to safety in the face of the contradictions between the risk models saying everything was fine vs. the actual losses indicating otherwise. The organizations that failed, in simple terms, assumed reality would eventually conform to the model. The rest is history.
4. A Tree Falls in the Forest (without a feedback loop)
Risk quantification has to exist in feedback loops (positive and negative). Risk quantification approaches need to be constantly refined so you can either build increasing confidence in the approach or that it can be discarded. There are well developed bodies of knowledge and practice to do this across a range of risk disciplines that can be tapped into that include: sense-making, comparability, managing model complexity, validation and back-testing models through to asserting and managing ALWs (Assumptions, Limitations and Weaknesses) of models. But the key to all of this is making sure there are one or more feedback loops in place to analyze how accurate the model is in the face of reality and under what conditions the risk quantification approach breaks down. This will guide where the approach should be used and in what way. These feedback loops, of model accuracy, are also only effective and useful if, of course, they are in turn placed in the context of the feedback loop of deploying control adjustments according to what the model is indicating.
5. All Risk Quantification is Wrong (but some is useful)
All risk quantification is wrong, but some is useful (paraphrased). When approaching risk quantification another mistake I’ve seen is for people to try and get too sophisticated too fast. In reality, some basic metrics like a key control indicator to simply measure what you expect can be remarkably effective, but only if you build the process around it to hold the environment to that. In many organizations, simply coming up with 20 or so top metrics that are emblematic of, or proxy for, risk in the environment are good enough. For example: if you can pick 20 metrics that encapsulate a number of the CIS Critical Controls and work like crazy to keep your environment to those then you will likely get more benefit than spending your time on more sophisticated approaches.
Avoid cute but inscrutable index based metrics that aggregate counts but don’t reveal the best courses of action. Think about it, how many “cybersecurity indexes” have you seen that initially you might think are useful and then you (or those you inflict it on) ask simple questions like, “What is one thing I can do to move this index the most for the least effort?”, “What is an acceptable value of this?”, or even “If I get below this value I won’t be breached right?......right?”
Instead, for example, think about bringing counts together in different ways, for example: rather than some index of control contribution to mitigate the risk of an external threat acting on some sensitive asset, simply come up with a control pressure metric that records how many layers of controls did it take to stop an attack. If attacks are mostly getting through the first few layers then that reveals some course of action is needed.
When you do need more advanced methods, because to use basic counts would be too complex and insufficiently forward looking, then be careful how you use them. Methods like FAIR are good, but they are best used in a macro way to influence broad decisions of resource allocation or prioritization vs. being used for every micro decision you might make.
If the cost of doing the risk analysis exceeds the cost of implementing the control then just implement the damn control.
This is especially true in a regime where you are raising the baseline to reduce the cost of control. As discussed earlier, the use of Bayesian Network models can be remarkably effective at simulating risk and control path analysis, especially when paired with Bow-tie approaches, to reveal some counter-intuition about which of your controls contribute most to mitigating a specific risk.
6. It's Multi-Disciplinary or Nothing
Risk quantification is a multi-disciplinary activity. Cyber risk, or technology risk quantification approaches should not exist in isolation. Some of the best inputs for cyber are from other risk disciplines in your organization whether it's something common to all organizations like data from your SRE or similar process through to more industry specific measures that are emitted from your compliance, safety, quality, financial risk, or scenario planning units.
Bottom line: we need to apply more quantitative risk analysis methods to cyber, but to think there will be one unifying approach is naive. Like every other discipline you will need to select the particular method to the task at hand and then iterate. Above all, don’t confuse risk communication techniques with risk quantification techniques. And remember, even when it’s all working your most important equation might well be Risk = Hazard + Outrage.
Comments